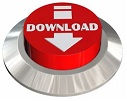
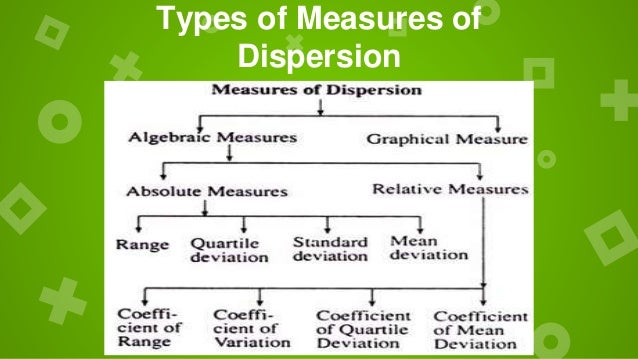
In the example above, the variance is 27. The variance is a measure of the dispersion, and its value is lower for tightly grouped data than for widely spread data. We call this averaging of the square of the differences from the mean (the mean squared deviation) the variance.

The sum of those squares is 216, so their average is 2168=27.
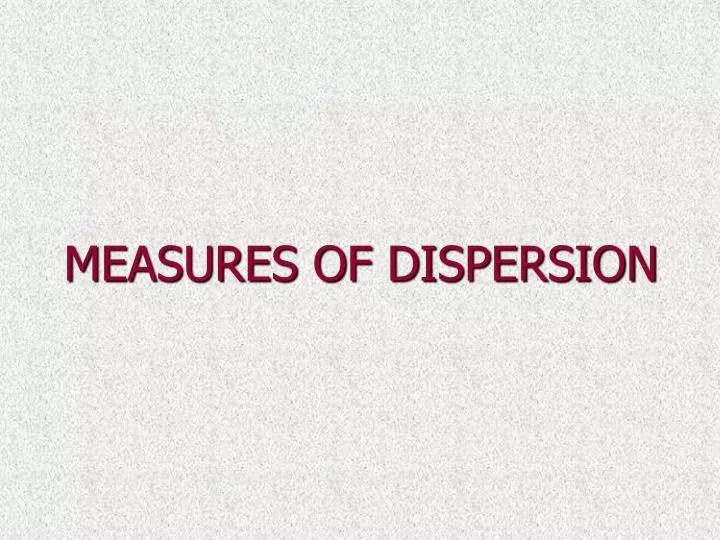
If we do that for this data set, we get the following list: So, the sum of the deviations is not a useful tool for measuring variance.īut if we square the differences, all the negative differences become positive, and then we can tell how great the average deviation is. You can use algebra to prove (try it!) that the sum of the deviations will always be zero, no matter what numbers are in the list. You can see that some of the values are positive and some are negative, as the mean lies somewhere near the middle of the range. If we take the mean of these deviations, we find that it is zero: Here’s a list of the values’ deviations from the mean: The mean of these values is 18 of course, the values all differ from 18 by varying amounts. A better method involves measuring the distance each data point lies from a central average. The range is not a particularly good measure of dispersion, as it does not eliminate points that have unusually high or low values when compared to the rest of the data (the outliers).
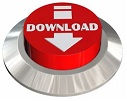